Recently we partnered with the EDM Council on a video that investigates the application of AI to data quality and matching.
In this EDM Talk, we lift the lid on how our AI team is developing solutions to help our clients, especially in the area of entity matching and resolution. This plays an important role in on-boarding, KYC and obtaining a single customer view.
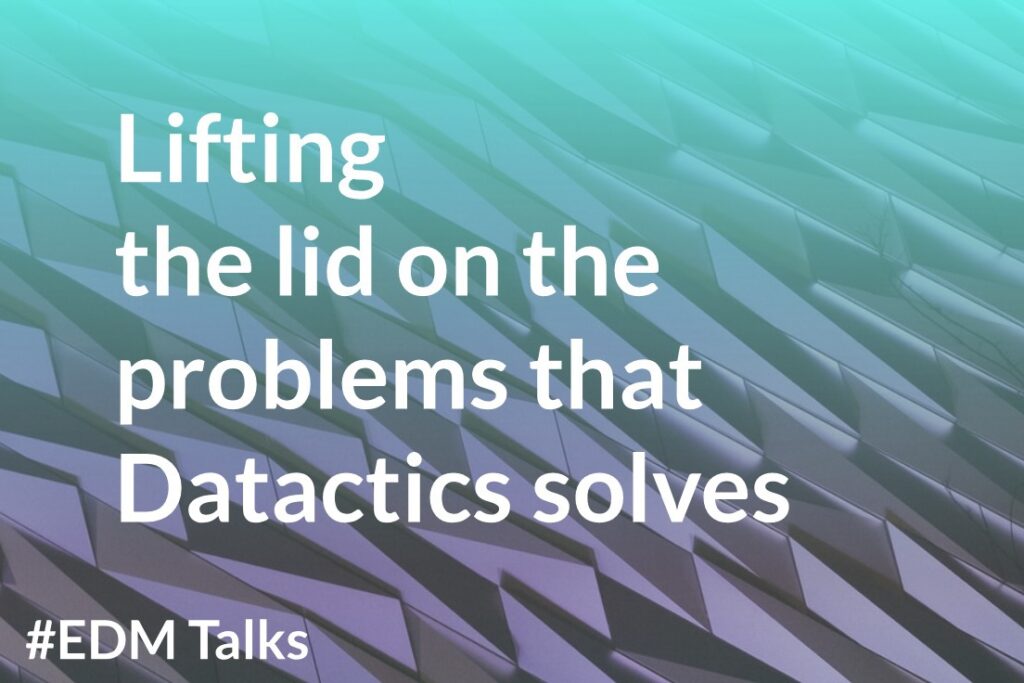
What is the the data challenge?
Institutions such as banks, often have large sets of very messy data which may be siloed and subject to duplication. When onboarding a new client or building a legal entity master, institutions may need to match clients to both internal datasets and external sources. These include vendors such as Dun and Bradstreet and Bloomberg, or taking data from a local company registration authority, such as Companies House in the UK. This data needs to be cleaned, normalised and matched to create a single golden record in order to verify their identify and adhere to regulatory compliance. For many institutions, this can be a heavily manual and time-consuming process.
What needs to be done to improve entity matching?
In entity resolution, there are two main challenges to address: the data matching side; and the manual remediation side which is required to resolve those instances where we have low confidence, mismatched or unmatched entities.
Datactics undertook a recent Use Case where we explored matching entities between two open global entity datasets Refinitiv ID and Global LEI. We augmented our fuzzy matching rule-based approach with ML to address and improve efficiencies around the manual remediation of low confidence matches. We performed matching of entities between these datasets using deterministic rules, as many firms do today. We followed the standard approach in place for many onboarding teams, whereby entity matches that are low confidence go into manual review. Within Datactics, data engineers were timed to measure the average time taken to remediate a low confidence match which could take up to one minute and a half per entity pair. This might be fine if there are just a few entities that you need to check but whenever you have hundreds, thousands or many hundreds of thousands this highlights how challenging the task becomes and the resource and time required to commit to this task.
At Datactics we thought this was an interesting problem to explore. We were keen to fully understand whether AI-enabled data quality and matching would bring benefits in terms of efficeincy and improvement to data quality to our clients who undertake such tasks.
What did Datactics want to achieve?
We were particularly interested to understand how we could reduce manual effort and increase the accuracy of data matching. We wanted to understand what benefits machine learning would bring to the process, using an approach that was transparent and which would make decision-making open and obvious to an audit or regulator.
What benefit is there from applying Machine Learning to this problem?
Machine learning is a broad domain. It covers application areas from speech recognition, understanding language to automating processes and decision making. Machine learning approaches are built on mathematical algorithms and statistical models. The advantages of these approaches is the ability of the algorithms to learn from data, uncover patterns and then use this learning to make predictions on new unseen cases. We see machine learning deployed in everyday life from our email filters through to personal assistance devices such as Amazon Echo and Apple Siri.
Within the financial sector, Machine Learning techniques are being applied to tasks including profiling behaviour for fraud detection; the use of natural language processing to extract information from unstructured text to enrich the Know Your Customer onboarding process; through to the use of chatbots to automatically address customer queries and customise product offerings.
At Datactics we view Machine Learning as a tool to automate manual tasks through to a decision making aid augmenting processing such as matching, error detection and data quality rule suggestion for our clients. This then frees up time and resource for clients enabling them to do more in their role.
How can machine learning be applied to the process of matching?
Within Datactics we have augmented our rules-based matching process with machine learning. Our solution has a focus on explainability and transparency to enable the tracing of why and how predictions have been made. This transparency is important to financial clients in terms of adhering to regulations through to the building of trust in the system which is providing these predictions. Using high confidence predictions, we can automate a large volume of manual review. For example, in the matching Use Case, we were able to reduce manual review burden by 45%, freeing up client’s time with expertise deployed to focus on the difficult edge cases.
At Datactics we train machine learning models using examples of matches and non matches. Over time patterns within that data are detected and this learning can be used to make predictions on new unseen cases. A reviewer can validate the predictions and feed this back into the algorithm. This is known as human in the loop machine learning. Eventually the algorithm will become smarter in predictions making more accurate predictions. High quality predictions can lead to less manual review, by reducing the volume that need reviewed.
The models we have built need good quality data. We used the Datactics self-service data quality platform to create good quality data sets and apply labels to that data. Moving forward at Datactics, we are seeking to augment AI and to look at graph linkage analysis, as well as furthering enhancing our feature engineering and data set capabilities.
To learn more about what the work we are doing with machine learning and how we are applying it into the Datactics platform, all content is available on the Datactics website. We also have a whitepaper on AI-enabled data quality.
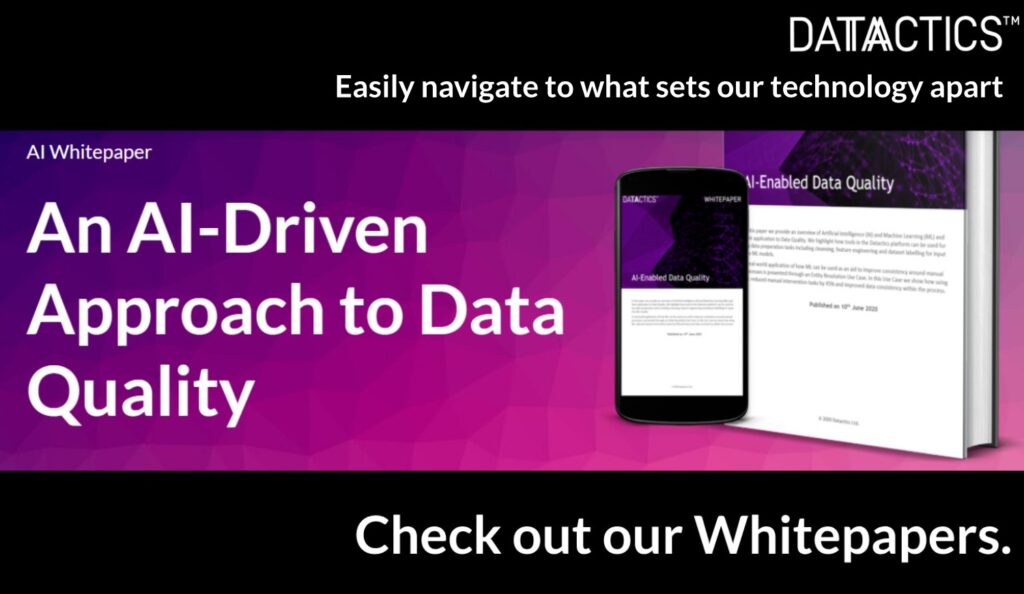
For a demo of the system in action please fill out the contact form.
To find out more about what we do at Datactics, check out the full EDM talks video below!
We will soon be publishing Part 2 of this blog series that will look at the application of AI and ML in the Fintech sector in more detail as well as an entity resolution use case.
Click here for the latest news from Datactics, or find us on Linkedin, Twitter or Facebook