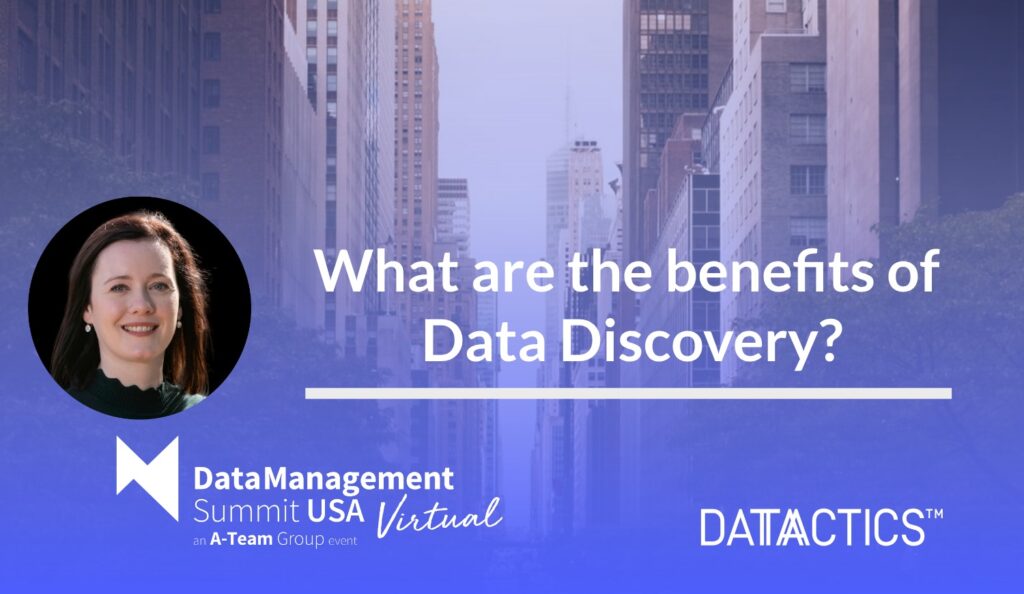
Datactics Head of AI, Fiona Browne, recently joined a panel at Data Management Summit USA Virtual discussing the science of data discovery and the art of implementation of business value. This panel sought to unpack the greatest data discovery challenges that have surfaced as a result of increased data volumes, and financial institutions making more use of cloud environments.
Fiona was joined by Brian Greenberg, Randall (Randy) Gordon, Linda Coffman, and Peggy Tsai.
The panellists focused on:
- The merits of leveraging external data management tools
- What exactly a robust DQ program or tool can do for your data
- The importance of creating a golden record or single version of the truth, and
- Implementing applications of AI/ML to automate some of the data discovery processes to generate better quality data across the enterprise.
Key takeaways from the discussions were:
- There are many benefits of data discovery
At Datactics, Fiona pointed out that we often see a spectrum of capabilities in terms of where institutions are in their maturity and data journey. Fiona stated that data discovery helps at all stages of implementing a Data Governance Framework, uncovering relationships and making use of things like metadata catalogs, but that at all times it is vital to understand the importance of embedding context to aid downstream applications and use cases.
Fiona said that it is vital to consider where you are with your Data Governance Framework, asking yourself the question: are you at the start of your journey, or are you well established within it? Do you have ownership and responsibility of the data, an understanding of security and accessibility; do you fully understand the regulations, legal implications and security of your data?
Data discovery is a key tool to be used at all times in these processes, enriching data with context that will help in the long run, whether mature or just starting out in Data Governance.
- Leading organisations are embedding a culture of data responsibility and understanding within an organisation.
These organisations have C-Suite executives right through to data engineers on the technical side all engaged on their roles and requirements when it comes to data as a prized business asset.
Firms looking to exploit this will need to focus on skilling up and training because this area is constantly changing, regulations are updating and adapting. For example, Fiona mentioned the new AI regulations from the EU that are coming down the line. This will require keeping an eye on best practices.
- Security is always moving!
Security is always moving and keeping up to date has never been more important. Accountability comes from assigning those roles and key responsibilities so that the key owner of this one single data state is known. There are many policies around data privacy, ethics and security, and of course quality so having a holistic view of those and then finishing off with best practices that then highlights well in terms of having your standardised data model across a company. One example is in building data catalogues, where it is important to categorise your data both in terms of risk and regulation.
- There is a wide range of tools and solutions that can help with data discovery
It depends on where you are on your data discovery and how much expertise and technology you have in house. Fiona stated there is a real range from data lineage and visualisation tools. There is also a lot of evidence that these tools are being augmented, for example, data lineage is being augmented with data quality metrics as it flows through an institution and technology to aid for example with metadata management.
The rise in ‘no-code’ platforms, such as Datactics’ Self-Service Data Quality, helps subject matter experts with things like measuring and monitoring their data quality, improving their data quality right through to things like graphs and graph analysis to uncover potential links and relationships between data as systems get larger and more complex by nature.
Fiona rounded off the discussion by stating that it is extremely encouraging to see us moving along in terms of augmenting the data discovery process with Machine Learning. This will address those more manual, time-consuming tasks that data stewards, analysts and business users end up saddled with.
About Self-Service Data Quality
Our no-code platform allows users to create complex rule logic with a drag and drop interface with no specialist programming skills required. Datactics Self-Service Data Quality platform empowers business users to self-serve for high-quality data, saving time, reducing costs, and increasing profitability.
In the highlight video below, you can watch Fiona discuss these areas in greater depth.