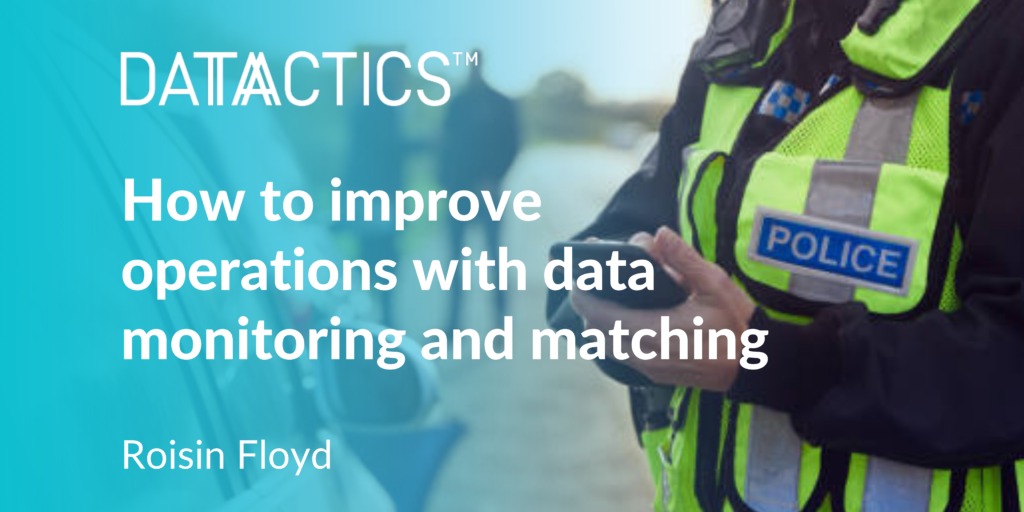
The National Digital Policing Strategy discusses some of the challenges the police are facing in terms of achieving digital transformation. Among them, legacy technology lock-in, conservative risk appetite and inconsistent understanding of data are listed as key barriers. In our experience, data quality monitoring and matching is key to achieving operational efficiency.
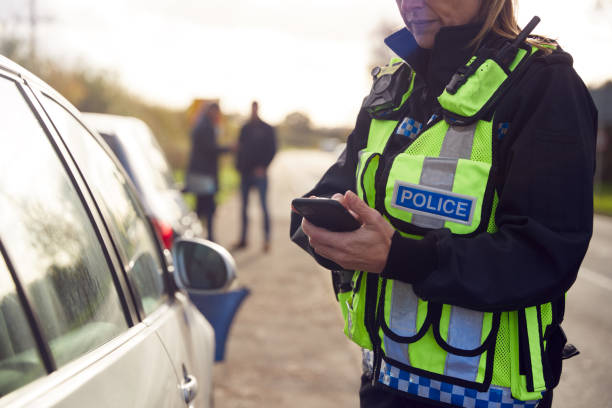
‘Policing in the UK remains world leading and sets the standard for law enforcement agencies across the world, however, our service is under pressure.’ (NPDS 2020-2030)
The challenge of ‘inconsistent understanding of data’ struck a chord with us. Given the amount of growing pressures on forces to deliver exceptional services and ensure compliance with regulations, it is essential that data is interpretable. In other words, that the data is clean, accurate and available. Access to high quality data is crucial for efficient decision making and problem solving; having messy data in different systems and silos is worse than having no data at all! This blog will look at two recommendations for police and public sector to use their data as an asset and achieve operational excellence.
Data Monitoring
As the saying goes, you can’t manage what you can’t measure. Using data quality metrics, police and other organisations can monitor their data to ensure it is up to date and compliant. The six core data quality dimensions- completeness, coverage, conformity, consistency, accuracy, duplication, timeliness, are good benchmarks for evaluating your data quality efforts. Not only are these guiding principles useful for continually improving your data, they also help to safeguard against compliance risks. For example, the timeliness of data is inherent in the RRD process, whereas validity and completeness are key to GDPR compliance.
Using automation eases the burden of manual monitoring of data for police forces. Automation can be used to monitor data that is subject to simple accuracy checks (e.g. postcode formats) or more complex rules around gazetteer data, improving data quality by continuously monitoring for rule defying data.
Data stewards can then review the improvement or degradation of data quality over time through a dashboard, enabling better data governance and strategic decision making. Datactics Self Service Data Quality platform automatically benchmarks data against a set of pre-determined rules.
Reliable, real world information underpins every aspect of efficient operational policing. When faced with outdated systems, data can end up being pulled from multiple sources, resulting in inefficient decision making and increased data quality issues. Adopting the practice of data preparation and monitoring can equip forces with the skills needed to achieve long term data integrity. Ultimately, utilising data-driven technology is a key enabler for protecting the public they serve.
Data Matching for Single Citizen View
Policing (and public sector more generally) can improve their key operations by using data matching to tackle crimes related to citizen data.
Data matching compares one data set against another, in order to get a single record of a citizen or customer (also known as a 360 degree view). Within the financial services industry, correlating disparate data into a summary of a customer allows for better understanding of their behaviours and circumstances. Insights into customer experience can benefit their business decisions and KYC efforts, with a clearer understanding of a customer’s risk potential, or their likelihood to buy new products.
Opportunities for change within Public Sector
In the same way, public services can benefit from a lean manufacturing approach to gain greater insights into citizen data. A common obstacle within public sector is merging multiple data sets stored in legacy systems. Without greater data sharing, justice and emergency services can suffer from a loss of critical insights.
A data matching exercise tackles this by starting with powerful matching on large data sets and de-duplication logic, allowing for highly configurable fuzzy matching on information such as names and addresses. The result is a single golden record or a series of candidate records rated for how closely they match. However, previous metadata is not ignored, as this is still useful in understanding a citizen’s past.
The automated nature of data matching tools highlights errors for further investigation, reducing the risks associated with missed matches and making it a regulatory imperative for public sector services. Pairing this capability with data quality tooling ensures that the standard of data is up to the level that digital transformation requires.
Tackling hidden crimes
Data matching offers benefits within policing. In policing, data matching can address issues around data falsification, where a suspect provides inaccurate information to avoid detection e.g. name or address. Moreover, it allows for maximized regulatory reporting and improved predictive analytics; transforming data from a liability to an asset.
The National Fraud Initiative (NFI) is a good example of data matching happening right now. Fraud accounts for 40% of all crimes in the UK and is one of the most enduring threats to the public purse. As the Counter Fraud Function says ‘fraud is a hidden crime, in order to fight it you have to find it’. Operated by the Cabinet Office, the NFI’s sole purpose is detecting and preventing fraud on a large scale, preventing £245 million cases of fraud and error between 2018-2020.
Digitally enabled crimes are getting more sophisticated, as shown by the National Policing Digital Strategy. To mitigate these risks, technology-driven strategies are helpful with decision making and optimising resource deployment across public services.
Data matching provides a cost-efficient solution for operations in public services. It can help identify potential risks at an early stage and deliver vital services as efficiently as possible.
Through a combination of measuring and monitoring data, organisations can achieve better data quality and operational excellence. Public sector organisations can benefit from the Shingo model’s guiding principles, which is to say, continuous improvement and a systematic approach to improving company culture. The first steps of investigating your data governance framework can help you benefit from these principles.
Improved police data quality is a cornerstone of a data management strategy that can deliver both operational excellence and a more reliable, trusted public serving body.
Roisin researches and writes on data management for Datactics.
And for more from Datactics, find us on Linkedin, Twitter, or Facebook.