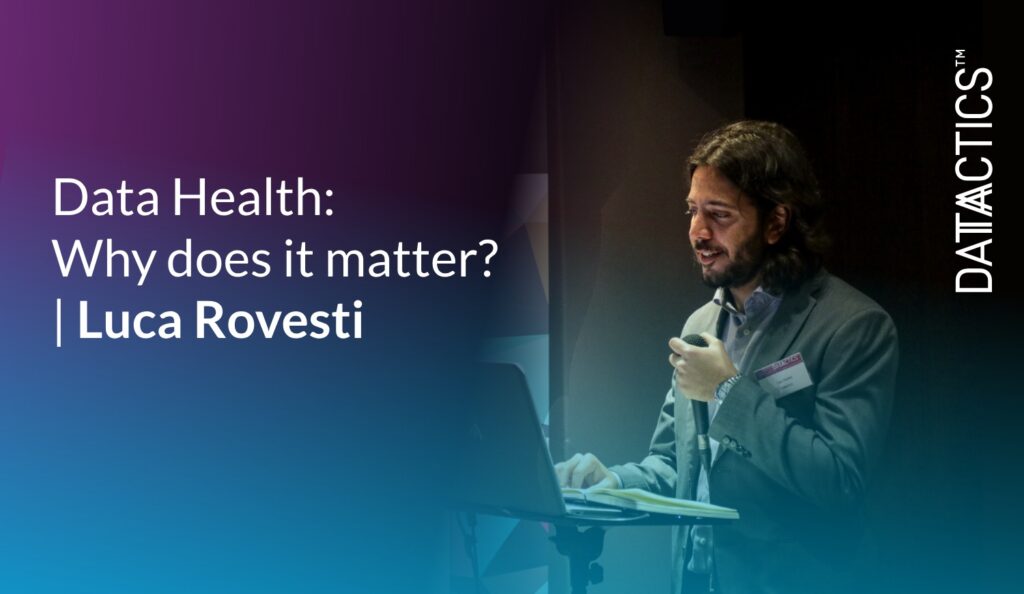
Introduction
Getting into shape is a big focus of summer advertising campaigns, where we’re all encouraged to eat, drink and do the right things to keep our bodies and minds on top form. You’ll be relieved to read that at Datactics we’re far more concerned about the health of your data, so open that packet of cookies, pour yourself another coffee, and let Luca Rovesti, Head of Product at Datactics, focus on your data wellness!
Why data health matters
With data being relied on more heavily than ever before, a clearer difference is emerging between being surrounded by data and using data to make business decisions. It makes sense then that these data needs will drive a corresponding greater need to keep that data healthy. It may be well known that healthy data means things like being complete, cleansed and compliant, but the problem is that the health of data is often not fully understood, and that this lack of understanding fuels a lack of trust. Trust in data is everything as more and more of the business is built on it.
To fully grasp the healthiness of your data, you must be able to prove the validity and completeness of that data. By achieving this, analytics can then be provided to key decision makers who can trust the data to make those critical business plans. In this blog, we will dig deeper into what’s required to achieve data health; how you can measure it; and the importance of having an understanding of what you are measuring.
Willpower needed!
Firstly, what do we mean by data wellbeing? Factors in personal wellbeing include social, emotional, and psychological wellbeing; in much the same way, in the data space you have tooling and strategy. And in the same way that in personal wellbeing you need to focus on these factors and commit to specific courses of action, you need to commit and execute on your data health strategy if you’re going to see a difference.
For instance, if the commitment is missing typically that means that tooling is brought in but not the right people are committed to make the data quality initiative work. Typically, what happens is that you fail to hit the target on the value-adds, the real-world business results that are ultimately relevant to the people you need to convince to pay for it and invest in it. You could have a bunch of data quality rules that you think make sense, but nobody really values the outcome of the rules; or you could have an implementation that does not follow the practices or the guidelines that the end-users of the data are willing to commit to. If it is difficult to get buy-in because you are trying to change their way of working, we would then align the DQ initiative with how the business users are already working, with the aim of giving valuable insight that makes sense in their existing context.
As with everything then, commitment is vital. One of the major differentiators that Datactics brings to the table is that we are not just about measurement, but also about remediation – doing something material and meaningful to the business with the metrics and the exceptions we have identified from the system. We are very often playing the role of a trusted partner; working alongside the business to enable them to make the necessary changes to data and to how they view it.
Ultimately, it’s about our client taking that step, armed with our support, technology, and coaching, to commit and fix the broken data. Some of our clients have taken the ‘carrot’ approach, for example publishing on an internal site the office with the best data quality compliance score across the whole of the UK. They hold metrics on how many exceptions have been remediated and they give an honourable mention to the ones that are doing something about them. Whereas other firms we work with are going down the ‘stick’ route, publishing metrics to point out those who are lagging behind on these types of initiatives. Either way, the point is that accountability of who is having to do something with the data drives the commitment to do something about it.
Knowing what to measure: it’s a business problem
One of the biggest risks – if not the biggest risk – in a data quality initiative is a disconnect emerging between the parties involved. On the one side there are people tasked with the project, to create metrics, and the technological solution that monitors that data. And on the other side, the people who are using that data and the purpose they have for it. It means that it’s critical to make the measurements relevant to why the data is being used. The only way to do that is to gain an understanding of what the data is, and the ultimate purpose for that data, so that the whole initiative can be aligned with that purpose.
So how can you measure data health?
It is important to remember that data quality is only one aspect of a data capability framework. There are some early steps required around the overall governance and infrastructure. Governance is more conceptual, where you need to have the logical framework on what you want to do with the data nailed down, and there is the practical aspect of doing something about it and that starts with having the infrastructure to do it. We, of course, are a technology solution, so we need hardware to be able to execute and we need the correct connectivity from our hardware to the various points that we need to measure, and then from there we can start to measure data health – in motion and on the various required points of the data journey. Our solution has a highly configurable rules-based approach, so these rules can be as granular or as business focused as the client wants. The metadata around the rules is there to describe the difference and what the rule is designed to measure. Almost inevitably then, there needs to be a reporting layer that displays metrics that are understandable from the point of view of somebody that is at executive/business level. The Datactics platform does just that, converting the rule results into business-driven areas such as critical data elements, regulatory compliance and source system scores. Combined, these give a strong insight into how the data quality relates to the business activities across the lines of business. It also gives the possibility of prioritising the resolution of the most critical issues. This makes data quality truly relevant to every business user from CEO to entry level.
Changing attitudes towards data health
As Datactics has been in this business for years, it’s reasonable to say we have developed a solid equation for continuous measurement of data quality and data health. Clients are now wanting to take the next step, and usually that next step is data health ‘in-motion’ – wherever the data flows throughout the enterprise. We now have all these metrics gathered by running this process over time, so now we must help the business establish what they can do with this data. For instance, helping them get insight into patterns showing how the data is being fixed. Clients are also talking about moving averages and measuring the time/effort required to correct an error as key indicators of a culture of healthy data.
The important thing to remember is that perfection doesn’t exist. Instead, there needs to be a concept of priority. Asking things like -why are you measuring data? Will a key rule impact downstream operation or will it ensure the data is in good shape for the future? What will make an operational difference that needs to be factored in to prioritising actions taken on the metrics? As clients are increasingly keen to take this next step, we are equally keen to help them achieve data health.
To continue this conversation, you can reach out to Luca, Head of Product at Datactics.
About Datactics
Our next gen, no-code toolbox is built with the business user in mind, allowing business subject matter experts to easily measure data to regulatory & industry standards, fix breaches in bulk and push into reporting tools, with full visibility and audit trail for Chief Risk and Data Officers.
If you want to find out more about how the Datactics solution can help you to automate the highly manual issue of data matching for customer onboarding, then please reach out to us! Or find us on Linkedin, Twitter or Facebook.