What is Data Quality and why does it matter?
Data Quality refers to how fit your data is for serving its intended purpose. Good quality data should be reliable, accurate and accessible.
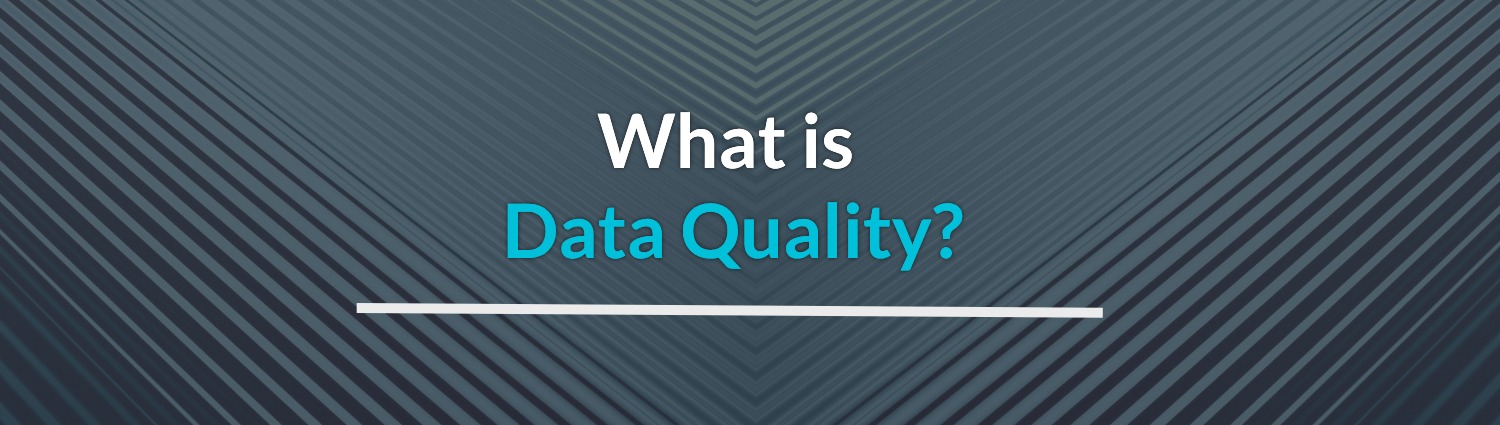
Good quality data allows organisations to make informed decisions and ensure regulatory compliance. Bad data should be viewed at least as costly as any other type of debt. For highly regulated industries such as government and financial services, achieving and maintaining good data quality is key to avoiding data breaches and regulatory fines.
As data is arguably the most valuable asset to any organisation, there are ways to improve data quality through a combination of people, processes and technology. Data quality issues can include data duplication, incomplete fields or manual input (human) error. Identifying these errors relies on human eyes and can take a significant amount of time. Utilising technologies can benefit an organisation to automate data quality monitoring, improving operational efficiencies and reducing risk.
These dimensions apply regardless of the location of the data (where it physically resides) and whether it is conducted on a batch or real time basis (also known as scheduling or streaming). These dimensions help provide a consistent view of data quality across data lineage platforms and into data governance tools.
How to measure Data Quality:
According to Gartner, data quality is typically measured against six main data quality dimensions, including – Accuracy, Completeness, Uniqueness, Timeliness, Validity (also known as Integrity) and Consistency.
Accuracy
Data accuracy is the extent to which data succinctly represents the real-world scenario and confirms with a source that is independently verified. For example, an email address incorrectly recorded in an email list can lead to a customer not receiving information. An inaccurate birth detail can deprive an employee of certain benefits. The accuracy of data is linked to how the data is preserved through its journey. Data accuracy can be supported through successful data governance and is essential for highly regulated industries such as finance and banking.
Completeness
For products or services completeness is required. Completeness measures if the data can sufficiently guide and inform future business decisions. It measures the number of required values that are reported – this dimension not only affects mandatory fields but also optional values in some circumstances.
Uniqueness
Uniqueness links to showcasing that a given entity exists just once. Duplication is a huge issue and is frequently common when integrating various data sets. The way to combat this is to ensure that the correct rules are applied to unifying the candidate records. A high uniqueness score infers minimal duplicates will be present which subsequently builds trust in data and analysis. Data uniqueness has the power to improve data governance and subsequently speed up compliance.
Timeliness
Data is updated with timely frequency to meet business requirements. It is important to understand how often data changes and how subsequently how often it will need updated. Timeliness should be understood in terms of volatility.
Validity
Any invalid data will affect the completeness of the data. It is key to define rules that ignore or resolve the invalid data for ensuring completeness. Overall validity refers to data type, range, format, or precision. It is also referred to as data integrity.
Consistency
Inconsistent data is one of the biggest challenges facing organisations, because inconsistent data is difficult to assess and requires planned testing across numerous data sets. Data consistency is often linked with another dimension, data accuracy. Any data set scoring high in both will be a high-quality data set.
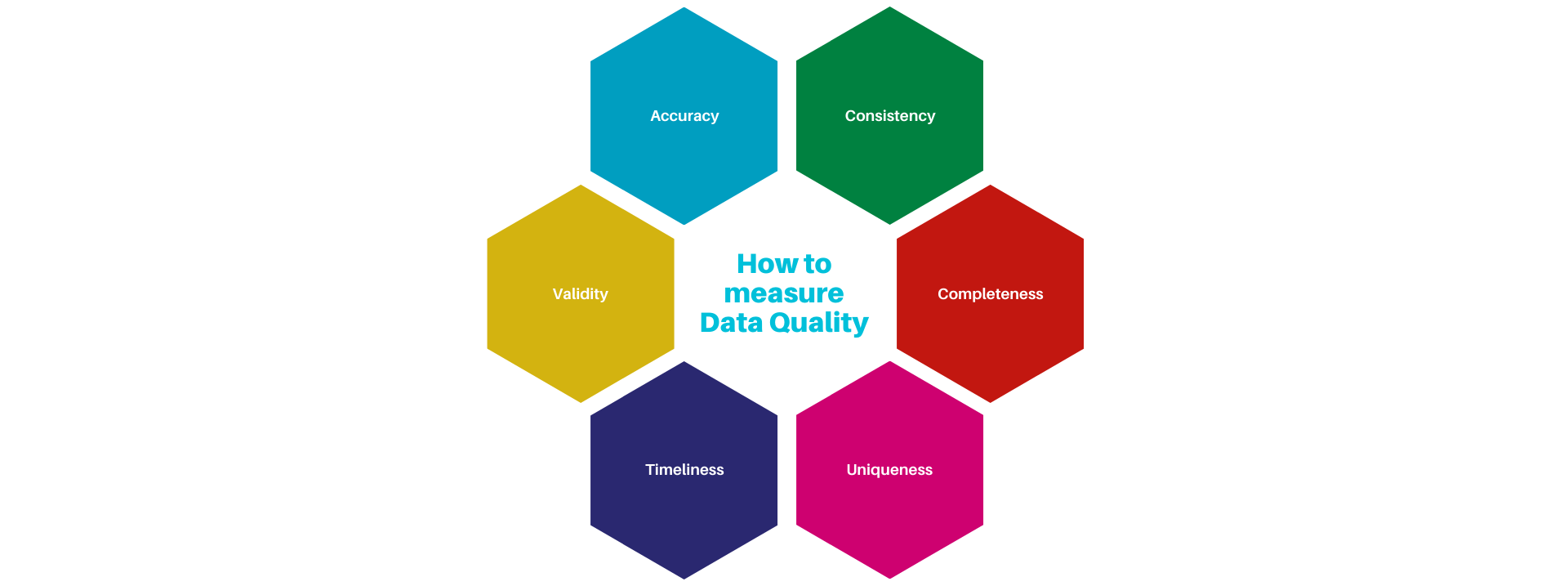
How does Datactics help with measuring Data Quality?
Datactics is a core component of any data quality strategy. The Self-Service Data Quality platform is fully interoperable with off-the-shelf business intelligence tools such as PowerBI, MicroStrategy, Qlik and Tableau. This means that data stewards, Heads of Data and Chief Data Officers can rapidly integrate the platform to provide fine-detail dashboards on the health of data, measured to consistent data standards.
The platform enables data leaders to conduct a data quality assessment, understanding the health of data against business rules and highlighting areas of poor data quality against consistent data quality metrics.
These business rules can relate to how the data is to be viewed and used as it flows through an organisation, or at a policy level. For example, a customer’s credit rating or a company’s legal entity identifier (LEI).
Once a baseline has been established the Datactics platform can perform data cleansing, with results over time displayed in data quality dashboards. These help data and business leaders to build the business case and secure buy-in for their overarching data management strategy.
What part does Machine Learning play?
Datactics uses Machine Learning (ML) techniques to propose fixes to broken data, and uncover patterns and rules within the data itself. The approach Datactics employs is of “fully-explainable” AI, ensuring humans in the loop can always understand why or how an AI or ML model has reached a specific decision.
Measuring data quality in an ML context therefore also refers to how well an ML model is monitored. This means that in practice, data quality measurement strays into an emerging trend of Data Observability: the knowledge at any point in time or location that the data – and its associated algorithms – is fit for purpose.
Data Observability, as a theme, has been explored further by Gartner and others. This article from Forbes provides deeper insights into the overlap between these two subjects.
What Self-Service Data Quality from Datactics provides
The Datactics Self-Service Data Quality tool measures the six dimensions of of data quality and more, some of which include: Completeness, Referential Integrity, Correctness, Consistency, Currency and Timeliness.
Completeness – The DQ tool profiles data on ingestion and gives the user a report on percentage populated along with a data and character profiles of each column to quickly spot any missing attributes. Profiling operations to identify non-conforming code fields can be easily configured by the user in the GUI.
Referential Integrity – The DQ tool can identify links/relationships across sources with sophisticated exact/fuzzy/phonetic/numeric matching against any number of criteria and check the integrity of fields as required.
Correctness – The DQ tool has a full suite of pre-built validation rules to measure against reference libraries or defined format/checksum combinations. New validations rules can easily be built and re-used.
Consistency – The DQ tool can measure data inconsistencies via many different built-in operations such as validation, matching, filtering/searching. The rule outcome metadata can be analysed inside the tool to display the consistency of the data measured over time.
Currency – Measuring the difference in dates and finding inconsistencies is fully supported in the DQ tool. Dates is any format can be matched against each other or converted to posix time and compared against historical dates.
Timeliness – The DQ tool can measure timeliness by utilizing the highly customisable reference library to insert SLA reference points and comparing any action recorded against these SLAs with the powerful matching options available.
Our Self-Service Data Quality solution empowers business users to self-serve for high-quality data, saving time, reducing costs, and increasing profitability. Our Data Quality solution can help ensure accurate, consistent, compliant and complete data which will help businesses to make better informed decisions.