What are Large Language Models (LLMs) and GPTs?
In today’s rapidly evolving digital landscape, two acronyms have been making waves across industries: LLMs and GPTs. But what do these terms really mean, and why are they becoming increasingly important?
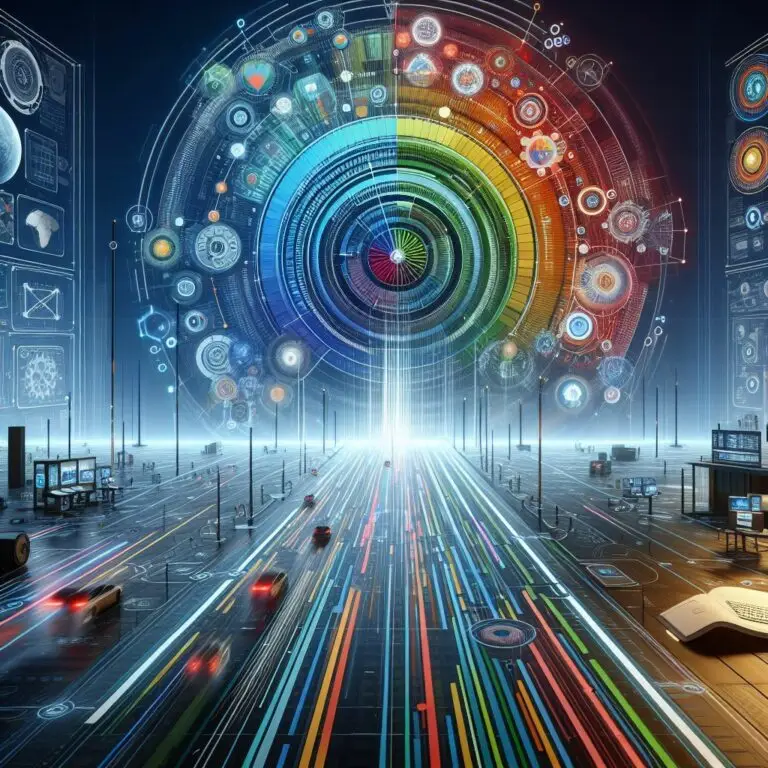
What are Large Language Models (LLMs) and GPTs?
As the digital age progresses, two terms frequently emerge across various discussions and applications: LLMs (Large Language Models) and GPTs (Generative Pre-trained Transformers). Both are at the forefront of artificial intelligence, driving innovations and reshaping human interaction with technology.
Large Language Models (LLMs)
LLMs are advanced AI systems trained on extensive datasets, enabling them to understand and generate human-like text. They can perform tasks such as translation, summarisation, and content creation, mimicking human language understanding with often remarkable proficiency.
Generative Pre-trained Transformers (GPT)
GPT, a subset of LLMs developed by OpenAI, demosntrates exactly what can be done with the capabilities of these models in processing and generating language. Through training on a wide range of internet text, GPT models are capable of understanding context, emotion, and information, making them invaluable for various applications, from automated customer service to creative writing aids.
The Intersection of LLMs and GPTs
While GPTs fall under the umbrella of LLMs, their emergence has spotlighted the broader potential of language models. Their synergy lies in their ability to digest and produce text that feels increasingly human, pushing the boundaries of machine understanding and creativity.
The Risks of LLMs and GPTs
Quite apart from the data quality-specific risks of LLMs, which we go into below, there are a number of risks and challenges facing humans as a consequence of Large Language Model development, and in particular the rise of GPTs like ChatGPT. These include:
- A low barrier to adoption: The incredible ease with which humans can generate plausible-sounding text has created a paradigm shift. This new age, whereby anyone, from a school-age child to a business professional or even their grandparents, can write human-sounding answers on a wide range of topics, means that the ability to distinguish fact from fiction will become increasingly complex.
- Unseen bias: Because GPTs are trained on a specific training set of data, any existing societal bias is baked-into the programming of that GPT. This is necessary, for example, when developing a training manual for a specific program or tool. But it’s riddled with risk when attempting to make credit decisions, or provide insight into society, if the biases lie undetected in the training dataset. This was already a problem with machine learning before LLMs came into being; their ascendency has only amplified the risk.
- Lagging safeguards and guardrails: The rapid path from idea to mass adoption for these technologies, especially with regard to OpenAI’s ChatGPT, has occurred much faster than company policies can adapt to prevent harm, let alone regulators acting to create sound legislation. As of August 2023, ZDNet wrote that ‘75% of businesses are implementing or considering bans on ChatGPT.’ Simply banning the technology doesn’t help either; the massive benefits of such innovation will not be reaped for some considerable time. Striking a balance between risk and reward in this area will be crucial.
High-quality data is the backbone of effective LLMs and GPTs. This is where Datactics’ Augmented Data Quality comes into play. By leveraging advanced algorithms, machine learning, and AI, Augmented Data Quality ensures that the data fed into these models is accurate, consistent, and reliable. This is crucial because the quality of the output is directly dependent on the quality of the input data. With Datactics, businesses can automate data quality management, making data more valuable and ensuring the success of LLM and GPT applications.
Risks of Do-It-Yourself LLMs and GPTs in Relation to Data Quality
Building your own LLMs or GPTs presents several challenges, particularly regarding data quality. These challenges include:
- Inconsistent data: Variations in data quality can lead to unreliable model outputs.
- Bias and fairness: Poorly managed data can embed biases into the model, leading to unfair or skewed results.
- Data privacy: Ensuring the privacy of the data used in training these models is crucial, especially with increasing regulatory scrutiny.
- Complexity in data management: The sheer volume and variety of data needed for training these models can overwhelm traditional data management strategies.
Conclusion
The development and application of LLMs and GPTs are monumental in the field of artificial intelligence, offering capabilities that were once considered futuristic. As these technologies continue to evolve and integrate into various sectors, the importance of underlying data quality cannot be overstated. With Datactics’ Augmented Data Quality, organisations can ensure their data is primed for the demands of LLMs and GPTs, unlocking new levels of efficiency, innovation, and engagement while mitigating the risks associated with data management and quality.